Improving the availability of rental bikes through model-based predictions of local demand
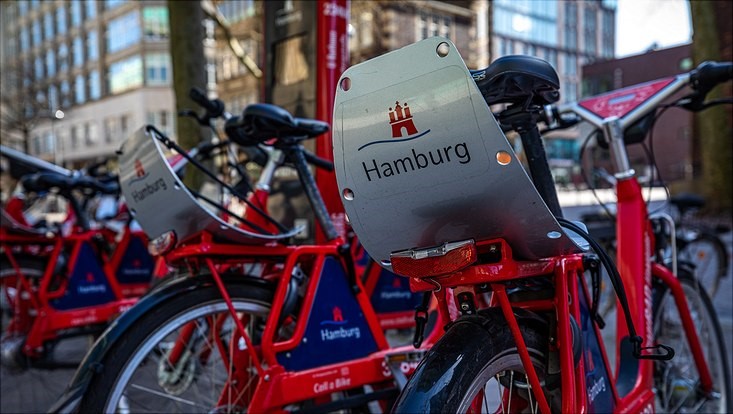
UHH/Ohme
In the Available Citybikes (Ava-Citybike) project, we want to improve the availability of rental bikes with the help of artificial intelligence. To this end, we are developing a neural network that predicts the local demand for rental bike stations. We are using this knowledge to achieve several goals.
On the one hand, we want to enable users to use rental bikes as a reliable means of transportation in everyday life. Due to local peaks in demand, rental bikes are currently temporarily unavailable at some stations. This is a major problem, as users rely on the availability of rental bikes every day to get to work, university or a leisure event. We will therefore use the predicted demand to enable users to plan ahead with the availability of rental bikes.
On the other hand, our offer is aimed at providers of rental bikes. They can use the predicted demand for rental bikes to distribute rental bikes in the network of stations. By distributing rental bikes, local bottlenecks of rental bikes between stations can be balanced out. This is also an important factor in increasing the availability of rental bikes at all stations in the long term.
In this project, we are looking forward to working on an interdisciplinary basis in the fields of mobility, computer science and psychology. The fact that it makes a lot of sense for our project to work in an interdisciplinary way becomes clear when we look at the project plan below.
In the first phase of the project, we will analyze the numerous factors that influence the demand for rental bikes and consider the most relevant ones for the development of our forecast. Example factors include time of day, weather, local events and traffic conditions.
We plan to use three complementary methods for the analysis:
- It can be assumed that relevant factors in the past will also be good predictors of demand in the future. We are therefore planning a statistical dependency analysis between the data set of city bike use in the last 5 years and the other factors. The dependency analysis determines how strong the correlation of the individual factors was on the demand for city bikes. In other words, how important the respective factor was for demand. This allows us to make a statistical statement about relevant factors for our forecast.
- Secondly, we plan to conduct interviews with employees of the regional rental bike provider Stadtrad. In the interviews, we particularly want to find out which methods and technologies Stadtrad is currently already using to improve the availability of rental bikes.
- Thirdly, we plan to conduct a user study. The aim of this study is to gain a better understanding of the context in which rental bikes are used. For example, we are interested in developing a reliable forecast of how often city bike users use the service, for what purpose and how users plan the route to their destination.
In the next phase, we will design and implement the neural network based on the selected relevant factors. We will then train the neural network using the Urban Data Hub dataset. In an iterative process, we optimize and train the neural network in order to finally obtain statements about the demand for rental bikes per station with the best possible accuracy, taking into account the relevant factors.
In the final phase, we will focus on implementing the neural network in one or more use case(s). In the project period of the next 12 months, we will weigh up which implementation is feasible, meaningful and expedient. The determination of the following three use cases is firmly planned in this phase:
- When navigating with Google Maps, for example, the forecast of the availability of a rental bike is displayed in the predictive route planning. Even with multi-modal routes - for example, a connection with a 30-minute train journey and then changing to a rental bike - it is very relevant for users to be informed early on about the availability of a rental bike after the train journey.
- On a city map of Hamburg, Stadtrad employees can see the predicted demand and improve local availability through distribution.
- The neural network is provided as an interface on the City of Hamburg's Urban Data Hub and serves as a data source for implementation in other services.
And here is the student group in their own words (German):
Studierendenprojekt: Verbesserung der Verfügbarkeit von Leihfahrrädern durch modellgestützte Voraussagen der lokalen Nachfrage
Förderzeitraum: 01.10.2022 - 30.09.2023 (12 Monate)
Studierende: Sebastian Rühmann & Radmila Khramtseva
Mentor: Prof. Dr. Janick Edinger